This is the second in a series of articles exploring the current EDC landscape and the critical challenges shaping the industry’s evolution.
In our previous article, two of Viedoc’s experts discussed the industry’s key challenges and proposed ways to move forward—reimagining the way forward and thinking beyond traditional EDC systems.
In this article, we again consult Majd Mirza, Viedoc’s Chief Innovation Officer, and Binish Peter, a Technical Fellow at Viedoc, to share their insights into concrete solutions for the current challenges.
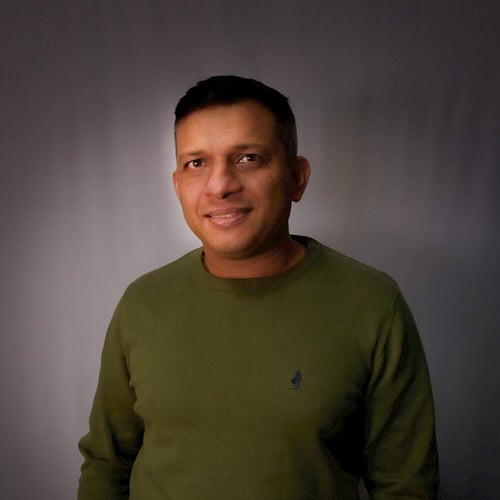
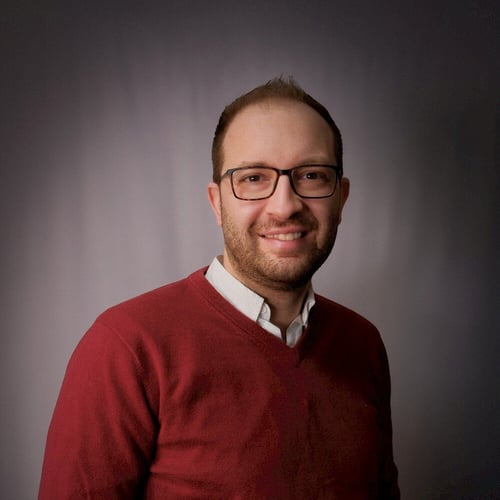
Setting the scene
Clinical trial protocols have become increasingly complex, leading to a significant increase in the volume of data collected (Tufts Center for the Study of Drug Development, vol 23 and vol 25). Today, we have numerous data sources, ranging from electronic data capture (EDC) and electronic patient reported outcome (ePRO) systems, to laboratories, imaging systems, electronic health records (EHR), and wearable devices. This shift necessitates the capture of continuous data streams rather than isolated data points.
Furthermore, data is sourced from and processed in different systems, making it essential to streamline data movement between them. The ultimate challenge is consolidating all this data into a single repository, enabling comprehensive aggregation and analysis within one unified system.
Evolving the platform
The transition from traditional EDC systems to comprehensive clinical data platforms is crucial for the success of future trials. EDC software must be re-engineered to collect data from diverse sources, aggregate, standardize, and store this information efficiently. They need to handle various formats and increased data volumes effectively while ensuring seamless availability to downstream systems.
How can we accomplish our infrastructure goals?
Two cloud techniques enable this transition—data pipelines and data lakes. Data pipelines streamline the collection, processing, and integration of data, ensuring a smooth flow of information. In contrast, data lakes address the challenges of volume, diversity, and aggregation of clinical trial data by providing scalable storage solutions.
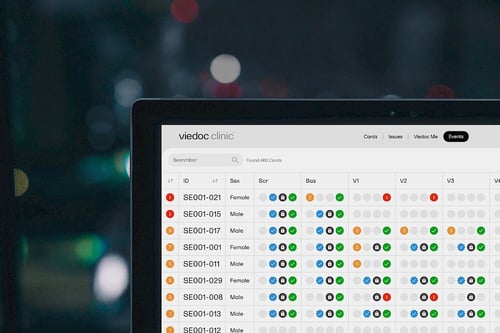
Data pipelines
Data pipelines, also known as ETL (extract, transform, load) pipelines, are designed to extract data from various sources, transform it into a standardized format, and load it into a destination system for storage or analysis. Leveraging cloud infrastructure, these pipelines manage tasks and dependencies between tasks, simplifying the process of extracting, transforming, and loading or storing data across systems.
Majd explains, “Azure provides Viedoc with customizable data pipelines. They can include various activities and be scheduled or triggered on demand. Think of them as a set of instructions or activities that direct the system: Where do I get the data from? What do I do with the data? How do I transform it? And what do I do with the result?”
Binish elaborates, “Data pipelines consist of a sequence of activities performed consecutively. Even though it’s called an ETL pipeline, the Viedoc clinical data platform doesn’t require all three components—extract, transform, load—to be in the same pipeline. We designed it to be flexible to accommodate varying requirements. In some cases, you need to extract, transform, and load data from one system to another. In others, you only need to extract and transform the data to enable access by other systems via API.”
Data lakes
Data lakes can store unlimited amounts of data in any format, whether structured or unstructured, in a single repository. They offer a scalable solution, capable of handling petabytes of data without compromising performance.
Each data or ETL pipeline activity can access the data lake, enabling the extraction, transformation, and storage of both raw and transformed data. This functionality allows for reanalysis, reaggregation, or reapplication of previous analyses while maintaining access to all original data.
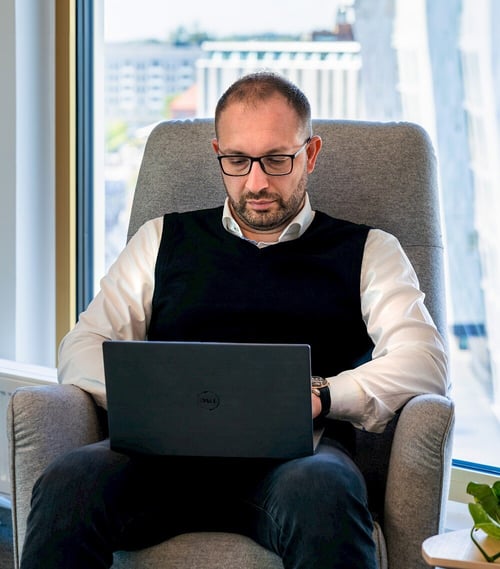
What differentiates Viedoc?
Viedoc offers flexibility by recognizing the need to gather data from multiple systems. To achieve this, custom connectors have been introduced to extract data from specific systems, such as popular wearables, and the system is designed to easily add other connectors over time.
Additionally, custom activities empower clinical data scientists to use their preferred programming languages, such as Python or R, to integrate with the data pipelines. This allows them to access real-time data for analysis, run AI modeling, or perform data cleaning in real-time using their scripts within the data pipelines.
Together, these components—ETL pipelines, data lakes, custom connectors, and custom activities—form the foundation of Viedoc’s new clinical data platform, which significantly diverges from traditional EDC systems. Unlike traditional EDC systems that focus on manual data entry of distinct data points and at set visits, our modern clinical data platform emphasizes automated data gathering from multiple sources and in real time, consolidating it into a single repository for comprehensive analysis.
Majd adds, “We aim to make this customizable platform affordable even for small and medium-sized companies. Our focus is on enabling easy no-code or low-code configuration of data movement and analysis, while still offering advanced coding capabilities for those who need it.”